Simply Explained: Monte Carlo Simulation for Risk Management
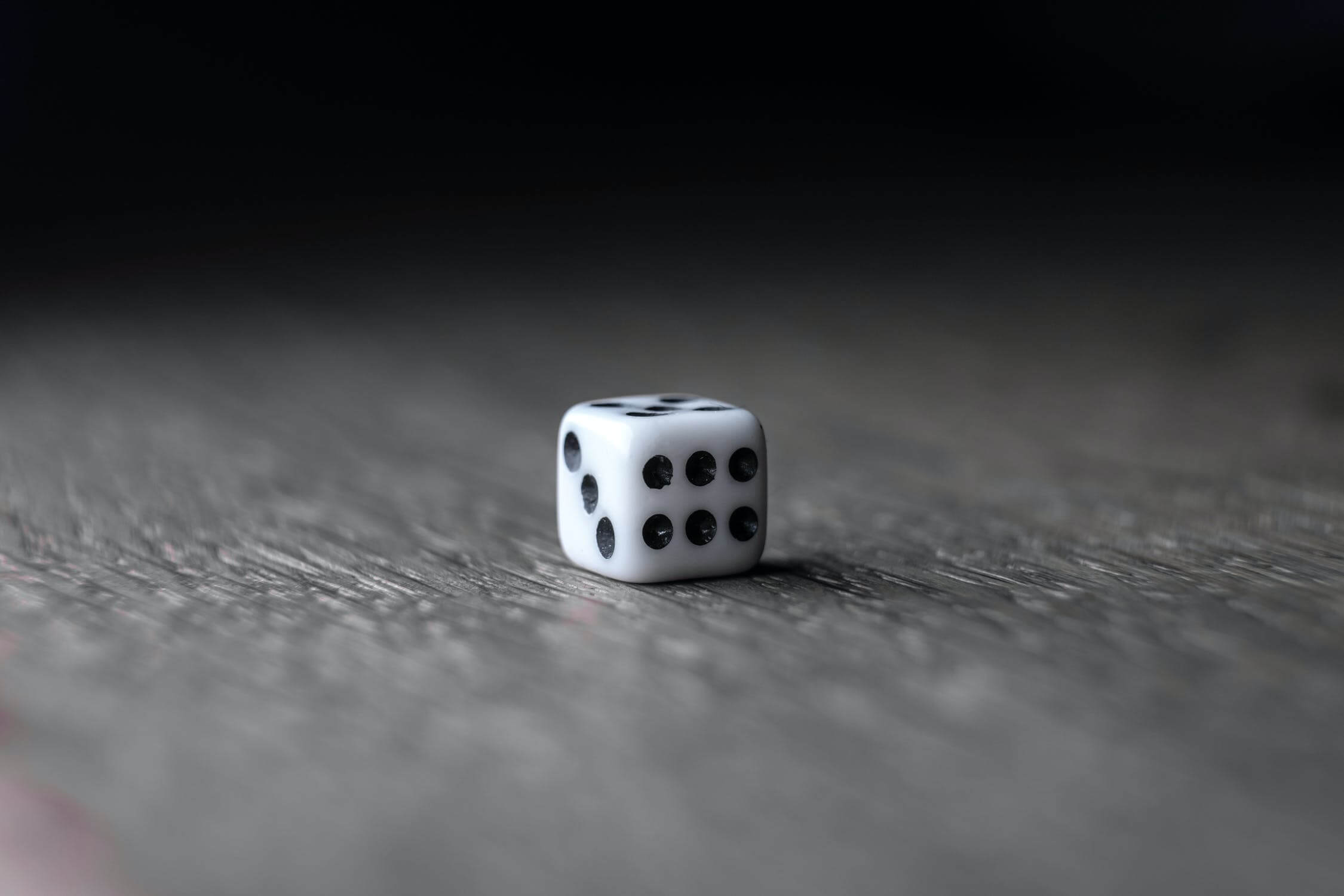
When it comes to decision making, we’re constantly faced with uncertainty and ambiguity. And even though we have almost unlimited access to information, we can’t predict the future accurately. That’s why risk analysis remains an inherent part of every decision we make.
Monte Carlo simulation – also known as Monte Carlo method – is used to understand the impact of risk in financial, project management and other forecasting models. It shows possible outcomes of your decision and allows to make better choices under uncertainty.
What is Monte Carlo simulation?
The modern version of the Monte Carlo method was invented in the late 1940s by Stanislaw Ulam while he was working on nuclear weapons projects. In 1946, physicists were investigating radiation shielding. Despite having most of the necessary data, physicists were unable to solve the problem using conventional, deterministic mathematical methods. Stanislaw Ulam suggested the idea of using random experiments.
Being secret, the work of Ulam required a code name. A colleague of Ulam suggested using the name Monte Carlo, which refers to the Monte Carlo Casino in Monaco where Ulam’s uncle would borrow money from relatives to gamble.
Today Monte Carlo simulation is widely used in the fields of finance, project management, energy, manufacturing, engineering, research and development, insurance, transportation, etc. The method is a computerised mathematical technique that shows the extreme possibilities (the outcomes of ‘risking it all’ as well as going for the most conservative decisions) along with all possible consequences for ‘middle-of-the-road’ decisions.
How Monte Carlo Simulation works
Monte Carlo simulation comes down to four simple steps:
#1. Identify a mathematical model of the activity or process you want to explore.
#2. Define the parameters for each factor in your model.
#3. Create random data according to those parameters.
#4. Simulate and analyse the output of your process.
Advantages of Monte Carlo Simulation
Monte Carlo simulation provides a number of advantages over deterministic, or “single-point estimate” analysis:
- Probabilistic Results. Results show not only what could happen, but how likely each outcome is.
- Graphical Results. It’s easy to create graphs of different outcomes and their chances of occurrence. This is important for communicating findings to other stakeholders.
- Sensitivity Analysis. It’s easy to see which variables had the biggest effect on the outcome.
- Correlation of Inputs. It’s possible to model interdependent relationships between variables and demonstrate how when some factors go up or down, others go up or down accordingly.
The Monte Carlo method allows to predict performance without conducting hundreds of experiments or building thousands of samples. In the modern world of limited resources – especially time – this is a powerful argument for giving the Monte Carlo method a try.